Soutenance de thèse de Ali Ballout
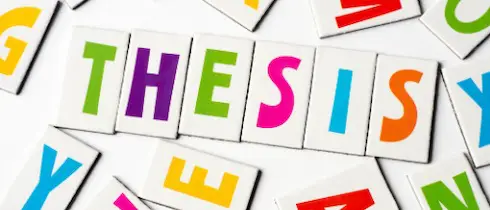
Ali Ballout presents her thesis defense on Monday 24 June at 2.00 p.m. The defense will be held on-line. The presentation will be in English.
The thesis entitled « Active Learning for Axiom Discovery » produced by the sparks division under the direction of Andrea Tettamanzi.
Abstract :
This thesis addresses the challenge of evaluating candidate logical formulas, with a specific focus on axioms, by synergistically combining machine learning with symbolic reasoning. This innovative approach facilitates the automatic discovery of axioms, primarily in the evaluation phase of generated candidate axioms. The research aims to solve the issue of efficiently and accurately validating these candidates in the broader context of knowledge acquisition on the semantic Web.
Recognizing the importance of existing generation heuristics for candidate axioms, this research focuses on advancing the evaluation phase of these candidates. Our approach involves utilizing these heuristic-based candidates and then evaluating their compatibility and consistency with existing knowledge bases. The evaluation process, which is typically computationally intensive, is revolutionized by developing a predictive model that effectively assesses the suitability of these axioms as a surrogate for traditional reasoning. This innovative model significantly reduces computational demands, employing reasoning as an occasional "oracle" to classify complex axioms where necessary.
Active learning plays a pivotal role in this framework. It allows the machine learning algorithm to select specific data for learning, thereby improving its efficiency and accuracy with minimal labeled data. The thesis demonstrates this approach in the context of the semantic Web, where the reasoner acts as the "oracle," and the potential new axioms represent unlabeled data.
This research contributes significantly to the field of automated reasoning, and beyond, opening up new possibilities in areas like bioinformatics and automated theorem proving. By effectively marrying machine learning with symbolic reasoning, this work paves the way for more sophisticated and autonomous knowledge discovery processes, heralding a paradigm shift in how we approach and leverage the vast expanse of data on the semantic Web.